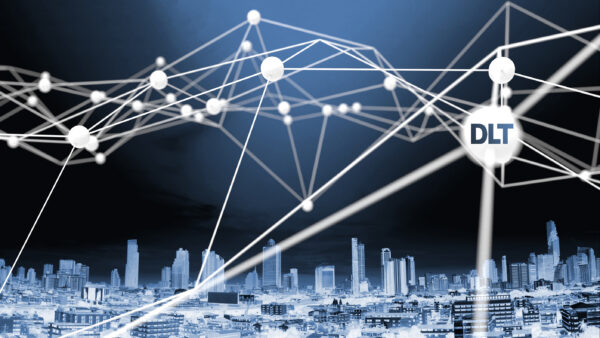
April 25, 2024
Regulated Liability Network: can the financial world ‘live as one’?
RLN will redefine digital marketplaces of the future, says R3’s Kate Karimson
Highlights
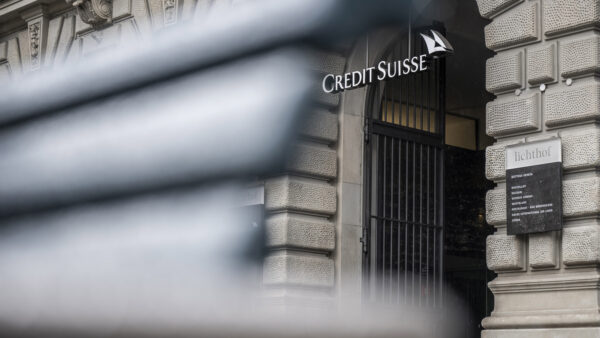
CoCo bonds have become too big to abolish
The alternative to AT1 debt would be extraordinarily costly for banks
Read more

April 24, 2024
Empowering CROs to succeed under the three lines of defence
The 3LOD model isn’t broken, but it remains a delicate balancing act
Read more
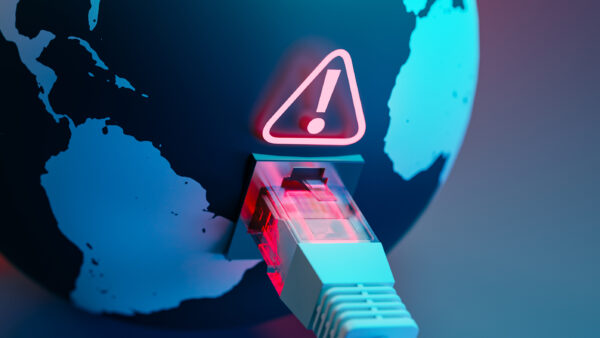
April 24, 2024
Hackers use developing countries as testing ground for new ransomware attacks
Hackers experiment in developing world before targeting businesses in the West
Read more
Continue reading
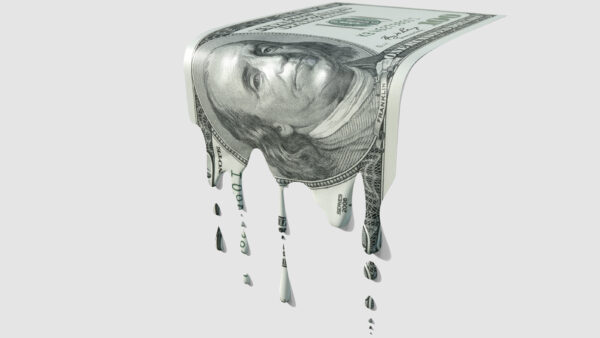
Liquidity saving solutions come to the fore
New Financial Stability Council consultation highlights need for 'liquidity preparedness'
Read more
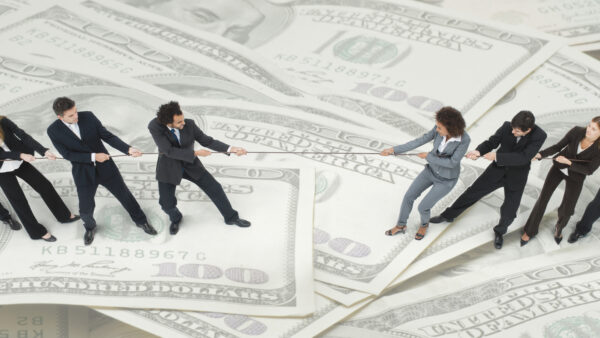
April 23, 2024
Bankers square up to regulators on merger reform
Radical proposals to shake up US bank takeovers are testing bankers’ patience
Read more
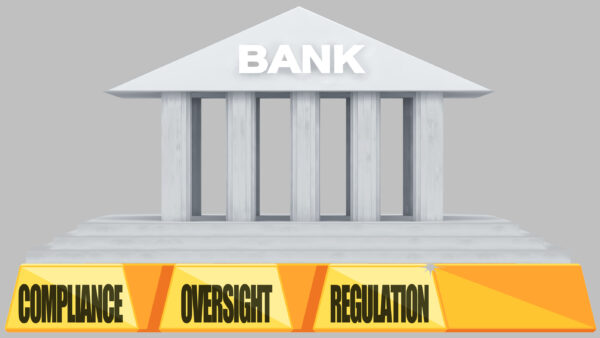
April 22, 2024
Is tougher supervision a free lunch for banks?
Why banks seeking long-term profitability might want to welcome tighter supervision
Read more
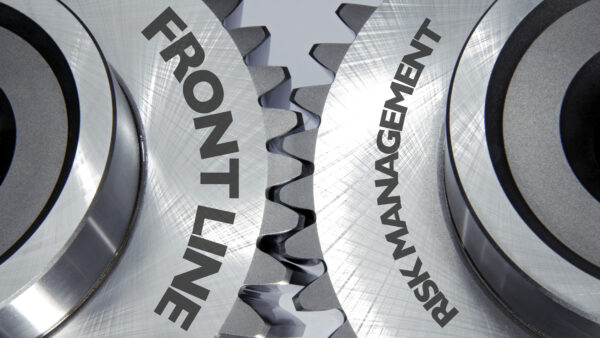
April 22, 2024
The risk function is not a ‘blocker’ but its image is
It’s time to improve the relationship between risk and the front line
Read more