
May 1, 2024
NBK’s French arm in ‘business blocking’ row amid staff turnover
Since its 2019 inception, the subsidiary has cycled through six CROs
Highlights
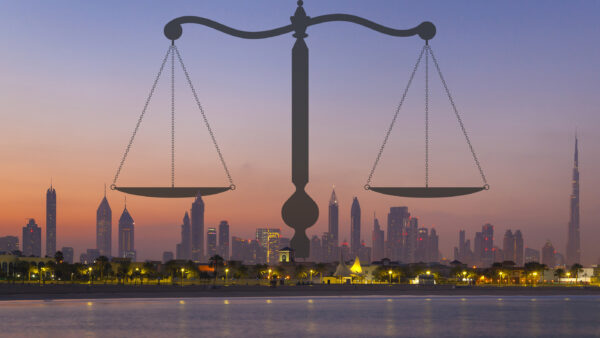
May 1, 2024
How the UAE can step up its fight against fincrime
Transparency on company ownership and stronger data analysis are obvious next steps
Read more

UK regulators take permissive AI stance
BoE and FCA to adopt principles-based, rather than prescriptive, AI approach
Read more

April 30, 2024
EU to give ‘unfiltered’ access to beneficial ownership information
European Parliament enshrines law to boost AML/CFT regime
Read more
Continue reading
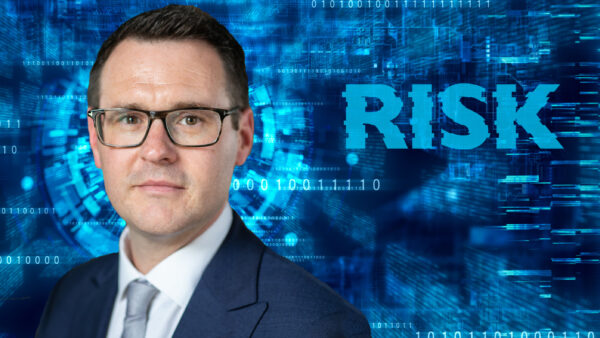
GenAI risk falls under OpRisk, ORX survey finds
Unified approach yet to emerge on who ultimately ‘owns’ GenAI risk
Read more

April 29, 2024
OakNorth hires new chief risk officer as it turns to US expansion
The incumbent and incoming CROs talk new business and going beyond 3LOD
Read more
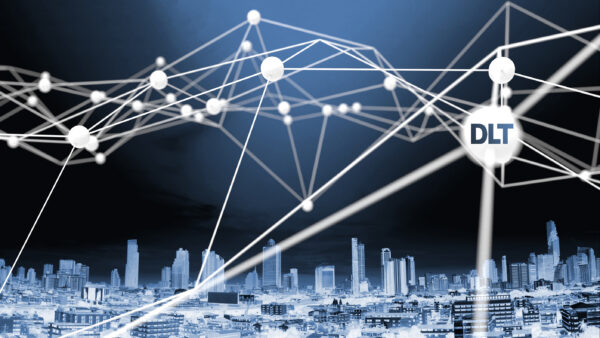
April 25, 2024
Regulated Liability Network: can the financial world ‘live as one’?
RLN will redefine digital marketplaces of the future, says R3’s Kate Karimson
Read more
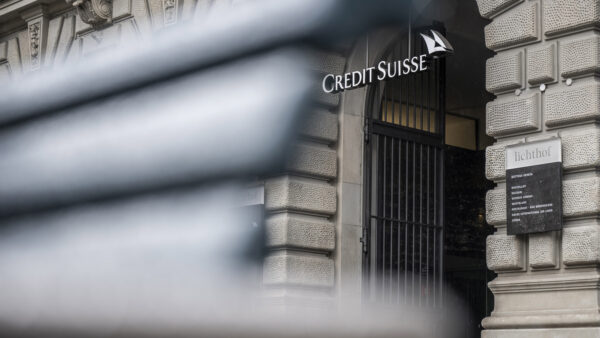
CoCo bonds have become too big to abolish
The alternative to AT1 debt would be extraordinarily costly for banks
Read more